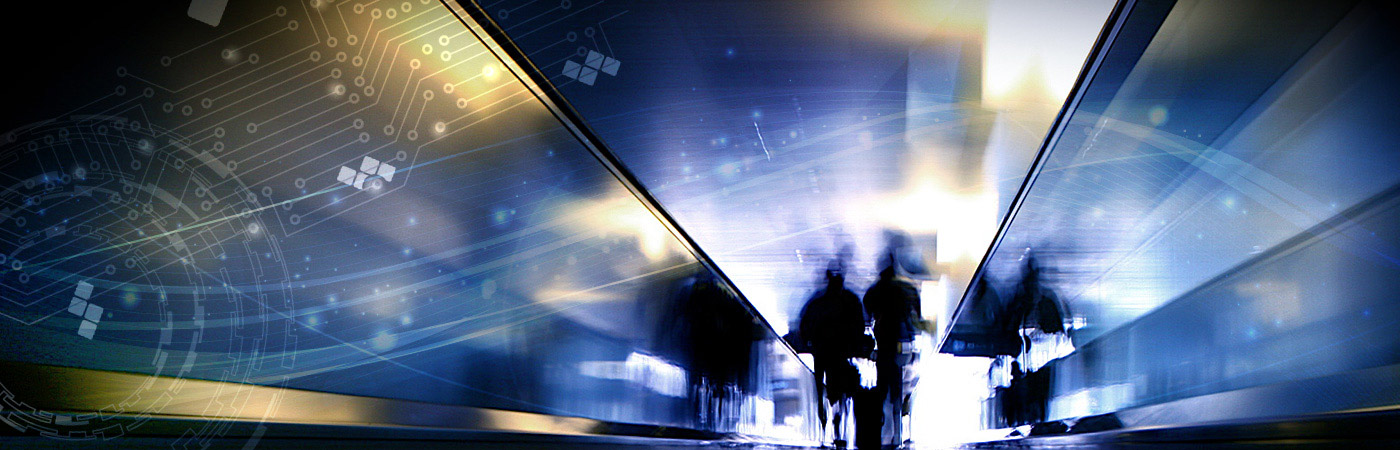
Alejandro Frangi
Bicentennial Turing Chair in Computational Medicine
What are your research interests?
My primary research interests lie at the crossroads of medical image analysis and modelling, machine learning (phenomenological models) and computational physiology (mechanistic models). I am particularly interested in statistical methods applied to population imaging and in silico clinical trials. My interdisciplinary work has been translated into cardiovascular, musculoskeletal and neurosciences.
What is the focus of your current research?
In-silico trials (IST) are computer-based medical device trials performed on populations of virtual patients. They use computer models/simulations to conceive, develop and assess devices with the intended clinical outcome explicitly optimised from the outset (a-priori) instead of tested on humans (a-posteriori). This will include testing for potential risks to patients (side effects) and exhaustively exploring in-silico for medical device failure modes and operational uncertainties before being tested in human clinical trials. Advanced computer modelling will prove helpful to predict how a device behaves when deployed across the general population or when used in new scenarios, reaching beyond the primary prescriptions (device repurposing) and helping to help the broadest possible target patient group without unintended consequences of side effects and device interactions.
What are some projects or breakthroughs you wish to highlight?
I hold a Royal Academy of Engineering Chair in Emerging Technologies in Computational Medicine, an emerging discipline devoted to developing quantitative approaches for understanding the mechanisms, diagnoses, and treatment of human disease through systematically applying mathematics, engineering, and computational science. Dealing with the extraordinary multi-scale complexity and variability intrinsic to human biological systems and health data demands radically new approaches compared to methods for manufactured systems.
A piece of work I am most proud of is a publication showing how in silico trials can replace the use of animals by accurately predicting the safety and efficacy of medical devices and drugs. Research into treating vascular diseases of the brain (e.g., aneurysm and stroke) typically uses large animals, including pigs, dogs and rabbits. Between 2000 and 2015, more than 430 rabbits and 160 dogs were used in studies focusing on the flow-diverting stents we modelled in silico. Virtual trials can provide evidence to ensure that only treatments with the highest likelihood of clinical benefit and lowest risk of complications progress to later-stage testing, avoiding using animals in preclinical safety studies. In addition, our in silico model goes beyond what is possible in conventional animal and human trials. Their virtual trials assessed an individual patient’s risk of complications and treatment failure, supporting clinical decisions to be made on a case-by-case basis. The model’s virtual population approach has broad potential applications across disciplines, such as cardiovascular (heart valves and pacemakers) and musculoskeletal (hip and knee replacements) medicine. The paper adds to the body of reliable regulatory evidence critical to support adopting in silico testing and trials in the UK and internationally. It was a key driver in establishing the in silico medicine innovation network (InSilicoUK), a community of scientists, learned societies and SMEs brought together by Innovate UK to advance the use of in silico approaches which replace the use of animals and improve patient outcomes. Check out a cool video we produce about this work.
What memberships and awards do you hold/have you held in the past?
I am a Turing Fellow and Fellow of the Royal Academy of Engineering, IEEE, SPIE and MICCAI. I also hold an ERC Advanced Grant and an IEEE Technical Achievement Award. Members of my created the start-up www.GalgoMedical.com in Spain, and I am founder and Chief Scientific Advisor of www.adsilico.uk.
What is the biggest challenge in Data Science and AI right now?
To ensure that the current and future workforce are ready to make the most of this digital revolution and we ensure this revolution does not leave people behind.
What real world challenges do you see Data Science and AI meeting in the next 25 years?
To move away from animal and human testing of medical products and ensure that virtual trials provide reliable regulatory evidence on safety and efficacy.
To develop self-adaptive and trustworthy learning systems that deal with uncertainty and imperfection in data without supervision.