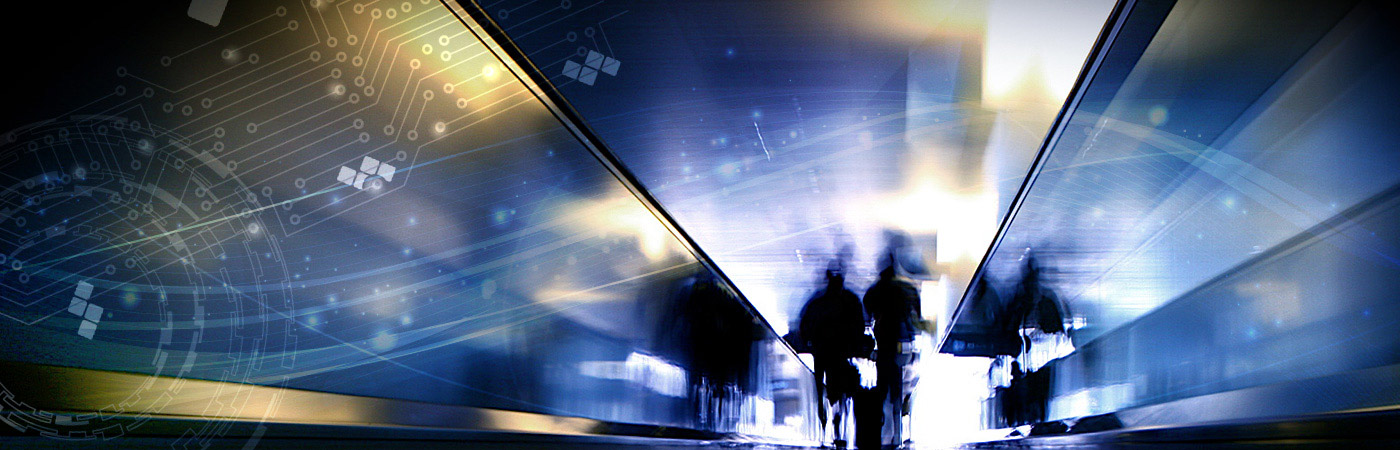
Magnus Rattray
Professor Computational & Systems Biology
What are your research interests?
I’m interested in discovering how biological systems work, and how they go wrong, by studying large, high-resolution biological and medical datasets. Biology is becoming an increasingly data-rich science, particularly with the introduction of technologies such as single-cell sequencing, spatial omics and high-resolution imaging. Machine learning and computational modelling can help us to make sense of these vast datasets.
What is the focus of your current research?
I recently obtained funding from the Wellcome Trust, with my colleague Hilary Ashe, for a major programme to study embryonic development at high spatial and temporal resolution. We aim to use cutting-edge spatial omics technologies combined with genetic perturbations and live cell imaging to learn gene-regulatory network models of embryonic development. This will help to better understand how the embryo develops in a way that is robust to external perturbations.
What are some projects or breakthroughs you wish to highlight?
In a recent paper leading to the above project we used a Gaussian process inference approach to infer messenger RNA degradation rates for hundreds of genes. This led to new insights into how the cell processes messenger RNA for degradation.
What memberships and awards do you hold/have you held in the past?
I’m a Fellow of the European Laboratory for Learning and Intelligent Systems (ELLIS) on the ELLIS Health Programme and Director of Manchester’s ELLIS unit. The aim of ELLIS is to strengthen AI research in Europe and ours is one of five ELLIS units in the UK. I’m the University’s academic liaison to the Alan Turing Institute and co-president of the Machine Learning for Computational Systems Biology COSI (community of special interest) of the main bioinformatics society ISCB.
What is the biggest challenge in Data Science and AI right now?
It’s challenging to integrate more traditional scientific modelling into data-driven machine learning approaches. During the pandemic we saw that most models being used were traditional mathematical models, such as differential equations, which are familiar tools in the scientific toolbox. By contrast, purely data-driven machine learning models can have great difficulty extrapolating into uncharted territory where there is little data available. Combining machine learning with other forms of modelling is beginning to happen, but I think we have a long way to go.
What real world challenges do you see Data Science and AI meeting in the next 25 years?
I think climate change is the biggest challenge facing us today and the world needs to move faster. It would be good to see data science and AI contributing to solving some major challenges in that area.