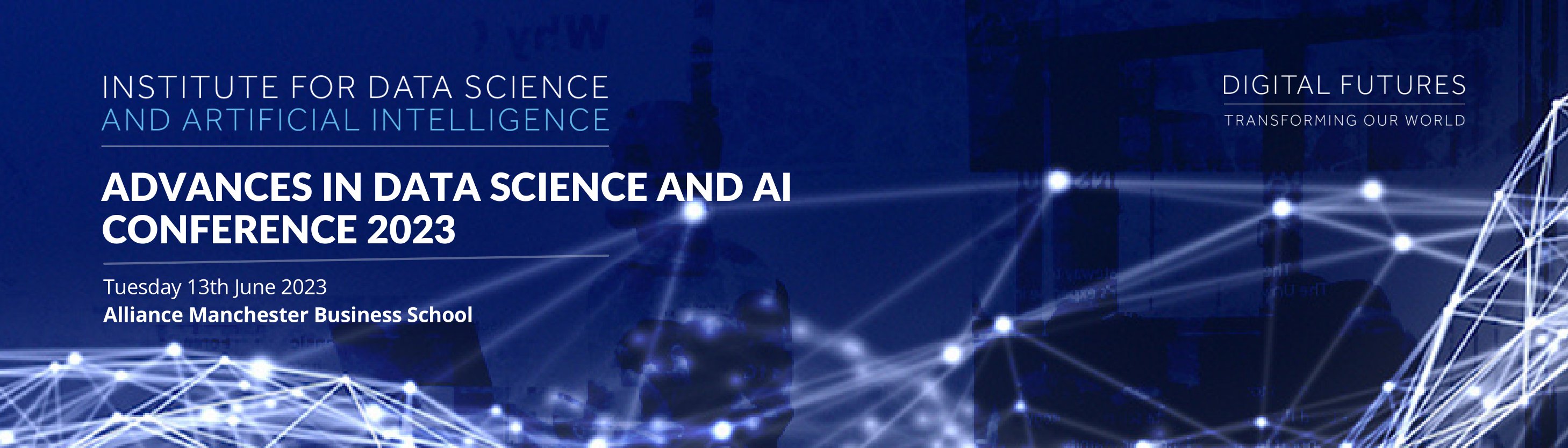
IDSAI | Advances in Data Science and AI Conference 2023
Following on from the success of ADSAI 2022, this year’s Institute for Data Science and AI’s annual Advances in Data Science and AI (ADSAI) Conference, a Digital Futures activity, took place at the Alliance Manchester Business School in Manchester on 13 June.
The Advances in Data Science and AI (ADSAI) Conference is Manchester’s annual data science and AI conference and brought together researchers from across the broad fields within data science and artificial intelligence to explore the latest advances and to discuss important topical questions being posed by society around the use of data science and AI.
The University of Manchester has been at the forefront of research in data science and AI since their inception. In the present day, we are expanding on this legacy with significant investments through large regional and international partnerships designed to advance research and nurture opportunities for growth in the Northwest of England.
This year we discussed the following topics:
- Digital Twins
- Human-Data Interaction
- AI for games
- Natural Language Processing
Conference Agenda:
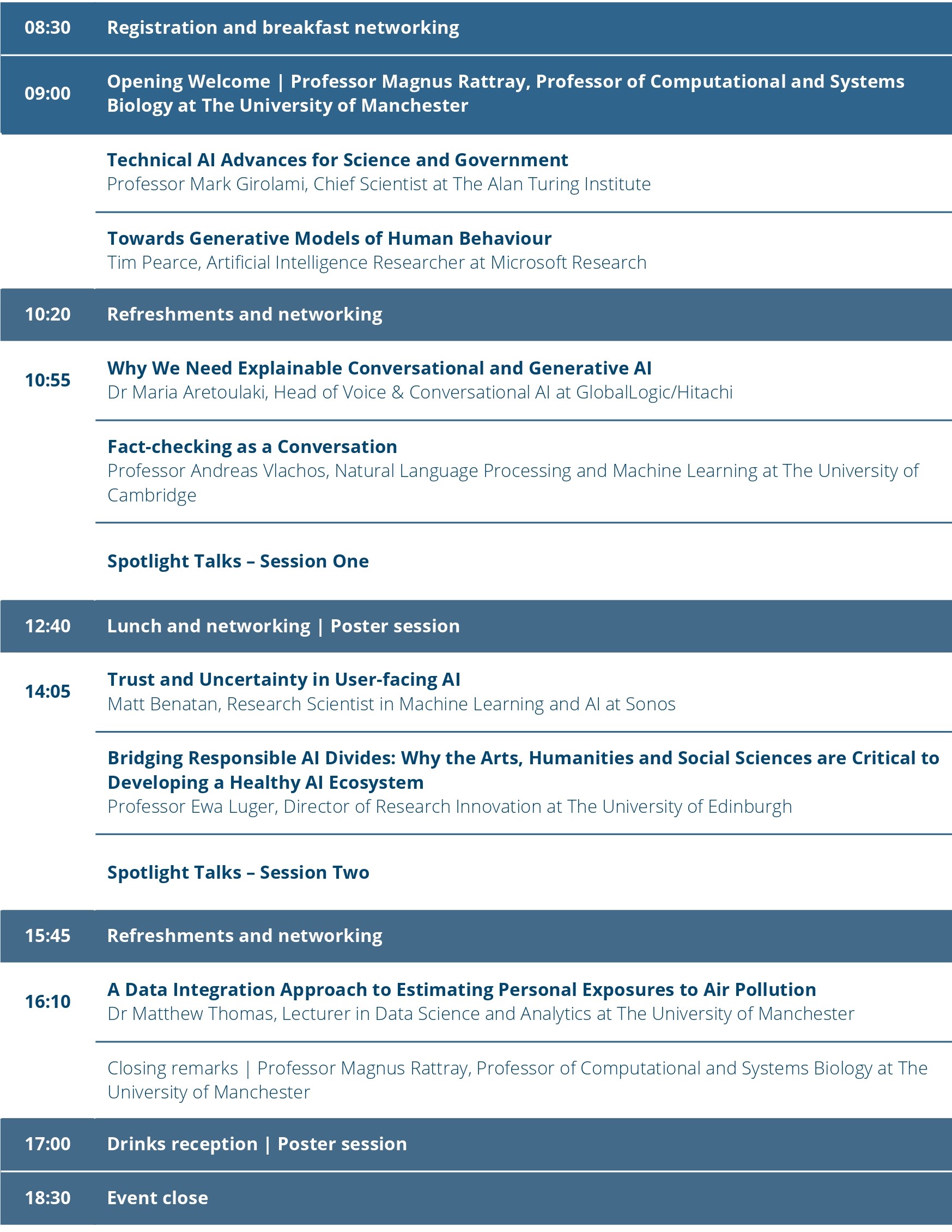
Conference Speakers:
Dr Matthew Thomas, Lecturer in Data Science and Analytics at the University of Manchester
A Data Integration Approach to Estimating Personal Exposures to Air Pollution
Air pollution is the largest environmental risk to public health and to develop and target mitigation strategies, there is a need to increase our understanding of the (personal) exposures experienced by different population groups. The Data Integration Model for Exposures (DIMEX) integrates data on daily travel patterns and activities with measurements and models of air pollution using agent-based modelling to simulate the daily exposures of different population groups. We present the results of a case study using DIMEX to model personal exposures to PM2.5 in Greater Manchester, UK, and demonstrate its ability to explore differences in time activities and exposures for different population groups. DIMEX can also be used to assess the effects of reductions in ambient air pollution and when run with concentrations reduced to 5 μg/m3 (new WHO guidelines) lead to an estimated (mean) reduction in personal exposures between 2.7 and 3.1 μg/m3 across population (gender-age) groups.
Professor Mark Girolami, Chief Scientist at The Alan Turing Institute
Technical AI Advances for Science and Government
The Alan Turing Institute is transforming itself and adopting a grand challenge-led approach to science and innovation. The institute recently released its strategy and announced Health & Medicine, Defence & Security, Environment & Sustainability as its three grand challenge priority areas. This talk will look at advances made at Turing in some of these priority areas, including: the assessment of Scottish Patients at Risk of Readmission, Seasonal Article sea ice forecasting with probabilistic deep learning, and the development and deployment of Ecosystems of digital twins.
Matt Benatan, Research Scientist in Machine Learning and AI at Sonos
Trust and uncertainty in user-facing AI
Over the past decade, machine learning has grown from a technical buzzword into a key component of our daily lives. An important contributor to its success is its impressive performance, which has inspired countless articles professing the superhuman capabilities of sophisticated deep learning models. But is media hyperbole sufficient to win over the masses?
We’ve all experienced both the best and worst that ‘smart’ technology has to offer, such as frustration with the smart thermostat, or elation when a music service discovers something we love. Ultimately, trust in AI comes down to day-to-day user experiences: the small, frequent interactions which underscore our relationship with technology.
Professor Ewa Luger, Director of Research Innovation at The University of Edinburgh
Bridging Responsible AI Divides: Why the Arts, Humanities and Social Sciences are Critical to developing a Healthy AI Ecosystem
The hype around the recent class of generative AI models such as Chat GPT, Midjourney and Crayon has captured the attention of governments, the media and the public. These models offer a low barrier to entry, allowing pretty much anyone with an internet-connected personal device to join the conversation, and with such democratisation comes risk. To mitigate this, we look to governments and regulators to protect our interests through the exercise of responsibility. This drive for responsible innovation has been motivated in large part by insights from the Arts, Humanities and Social Sciences and, if there was ever a time to shine a light on the importance of those disciplines, it is now. Bridging Responsible AI Divides (BRAID) is a new 3-year programme looking at precisely this. In this talk I will explore at some of the core challenges presented by data-driven innovations and present some of our early insights.
Dr Maria Aretoulaki, Head of Voice & Conversational AI at GlobalLogic/Hitachi
Speaker Bio:
Maria has been working in AI for Language Engineering for the past 30 years: NLP, NLU, Speech Recognition, Voice User Interface & Conversational Experience Design. Having started in Machine Translation and Text Summarisation using Neural Networks, she has focused on natural language speech and text interfaces for Contact Center automation and self-service. She has designed, built and optimised conversational Voicebots, Chatbots, Voice and Digital Assistants for large Enterprises worldwide (Big Tech, Telecoms, Retail Banks, Insurance, Utilities) and the UK Government, mainly through her own Consultancy, DialogCONNECTION. In 2018 she coined the term “Explainable VUI and Conversational Experience Design”, which later morphed to “Explainable Conversational AI” and more recently to “Explainable Generative AI” to advocate for transparent responsible design-led AI bot development that keeps the human in the loop and in control. She joined GlobalLogic / Hitachi at the start of 2022 where she has established and leads the Voice & Conversational AI Centre of Excellence and helps drive the company’s Generative AI strategy.
Talk Info:
Why we need Explainable Conversational and Generative AI
Chatbot technology democratisation and the ChatGPT hype has led to implementation arrogance and the current “AI Trump” plague. Terabytes of data and LLMs are not enough. We need to go back to basics: away from the extremes of frail hand-crafted but also completely unsupervised approaches, and towards a semi-supervised human-curated approach that leads to the golden duo of robustness and explainability. ‘Explainable Conversational and Generative AI’ combines data-driven Design with Human expert-curated Language and Speech Data Science. It ensures that we move away from pure processing (NLP) and random fact hallucination, to actual understanding (NLU) and the generation of accurate unbiased statements (NLG). This hybrid approach ensures optimal language coverage and at the same time the transparency, standardisation, customisation and user-centred control of a rule-based system.
Professor Andreas Vlachos, Natural Language Processing and Machine Learning at the University of Cambridge
Fact-checking as a conversation
Misinformation is considered one of the major challenges of our times resulting in numerous efforts against it. Fact-checking, the task of assessing whether a claim is true or false, is considered a key in reducing its impact. In the first part of this talk I will present our recent and ongoing work on automating this task using natural language processing, moving beyond simply classifying claims as true or false in the following aspects: incorporating tabular information, neurosymbolic inference, and using a search engine as a source of evidence. In the second part of this talk, I will present an alternative approach to combatting misinformation via dialogue agents, and present results on how internet users engage in constructive disagreements and problem-solving deliberation.
Tim Pearce, Artificial Intelligence Researcher at Microsoft Research
Towards generative models of human behaviour
Large generative AI models trained across massive diverse human datasets have had huge impact on the text and image domains. This talk considers how similar techniques can be applied to sequential decision making domains, as generative models of behaviour. Specifically, I’ll present recent work using diffusion models to imitate human demonstrations, showcased in video games and robotics. I’ll also reflect on the current status of reinforcement learning in the era of foundation models.
Conference organisers:
- Richard Allmendinger
- Mauricio A Alvarez
- Sophia Ananiadou
- Magnus Rattray
- David Topping
- Zhonghua Zheng
The Institute for Data Science and AI delivers the Data Science and AI theme within The University of Manchester’s Digital Futures platform.